Data sharing plays a vital role both in advancing scientific research and improving business success through the creation of data-driven product and service innovations. However, privacy concerns often limit the accessibility and utility of sensitive data. To address this challenge, synthetic data generation using Generative Adversarial Networks and Autoencoders has emerged as a promising approach. This paper presents a systematic literature review that explores the current research space of synthetic data sharing for privacy preservation. The systematic literature review encompasses methods, evaluation approaches, applications, domains, and scientific fields related to privacy-preserving synthetic data sharing. The vast majority of the selected 66 papers focus on sharing synthetic medical data, indicating a research gap for other domains. The findings of this study provide insights for Information Systems researchers to contribute to the advancement of Generative Artificial Intelligence for data ecosystems and highlight potential research directions.
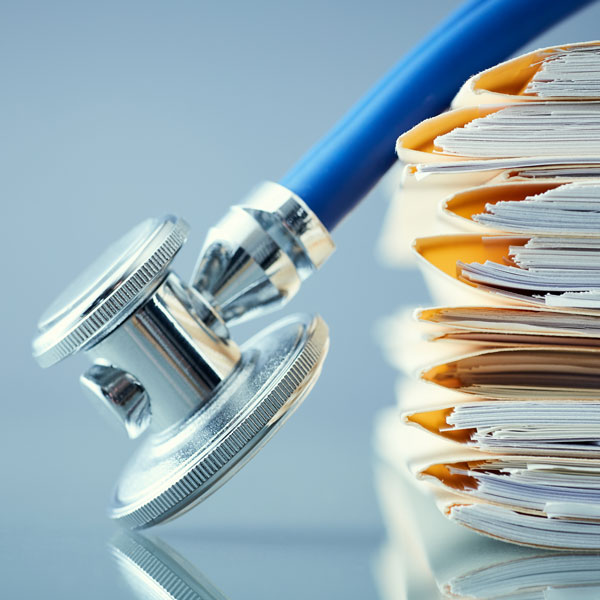